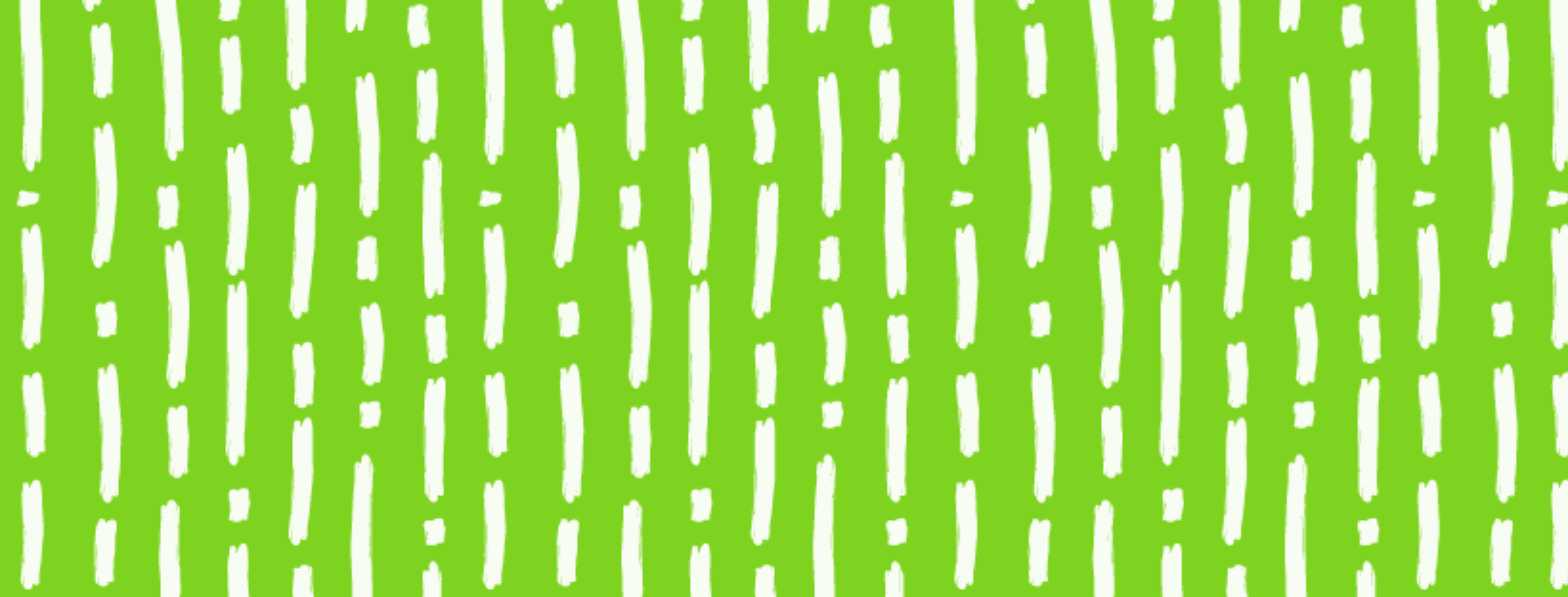
Papers
Fostering Data Collaboratives’ systematisation through models’ definition and research priorities setting
Federico Bartolomucci, Gianluca Bresolin. (2022)
Fostering Data Collaboratives’ systematisation through models’ definition and research priorities setting.
In Proceedings of the 23rd Annual International Conference on Digital Government Research (dg.o ’22).
Association for Computing Machinery, New York, NY, USA, 35–40.
https://doi.org/10.1145/3543434.3543442
Abstract
Data collaboratives (DC) [12, 18] have gained increasing attention in recent years benefitting and nurturing the momentum around the use of Data for Good [11]. However, research on the topic, derived and built upon the fields of collaborative governance, information sharing, and open data [9, 17] is still unmature, lacking a systematic body of knowledge, grounded in empirical evidence [11]. Except few studies, specifically referred to DC [31, 36, 37, 40, 42] [15, 18, 19, 24], most of the literature used in the field is encompassing broader concepts as such DataSharing, DataforGood, or Cross Sectoral Partnership.
Given that the empirical field has matured sufficiently to permit more quantitative analysis, the research seeks to go beyond existing qualitative classifications and inductively define data collaborative archetypes, emphasizing their distinctions and peculiarities as a foundation for future research on the topic.
The research started from a literature review on DCs, their definition and the dimensions identifying different DC’s models. The dataset provided on datacollaboratives.org has been filtered based on the literature review, excluding those instances that do not meet the DCs criteria or for whom online data collection is not feasible. Once the empirical setting was defined, a phase of variables selection and population has been conducted according to different variables. The evaluation of different clustering solutions, using both qualitative and quantitative methodologies, brought to identify five mutually exclusive clusters.
Each cluster is described according to 18 variables, allowing the emergence of cluster’s specific peculiarities and challenges. Findings are consistent with prior classifications and taxonomies [18, 29] with additional views afforded by a larger number of instances, the use of quantitative methodologies and the analysis of additional variables. Findings demonstrate the coexistence of quite different entities under the concept of DC, each of whose challenges and progress should be examined independently by researchers.
Responding to the objective to foster DCs long term sustainability, different research priorities are specified according to identified clusters and an empirical setting for conducting this research is made available. From a practitioner perspective, research’s findings may enable those interested in the topic to obtain more comprehensive information about benchmark examples, which is a valuable resource for industry growth. Additionally, the research illustrates the efficacy of categorical variable clustering analysis for inductive exploratory studies in a novel field of research.